Library
The following resources are courtesy of: University of Cambridge Royce Partner. Digital library assembly and maintenance funded via the EPSRC AI Hubs, AIchemy [aichemy.org] (EP/Y028775/1, EP/Y028759/1), APRIL [april.ac.uk] (EP/Y029763/1) and Royce (EP/…)
Ferromagnetic Materials
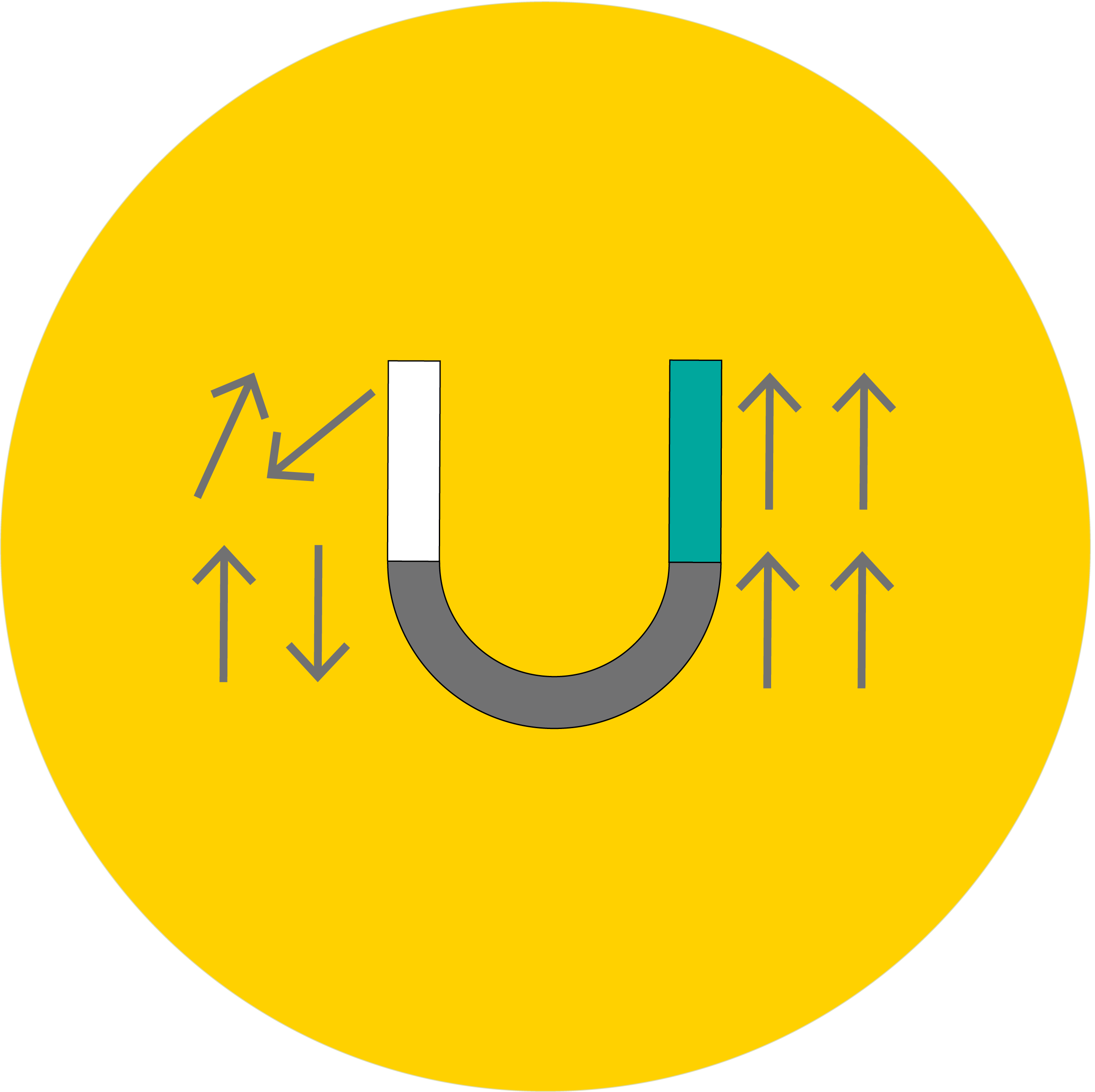
User Input: chemical composition of chosen material
Model Output: Curie temperature prediction
Licence: MIT
Superconducting Materials
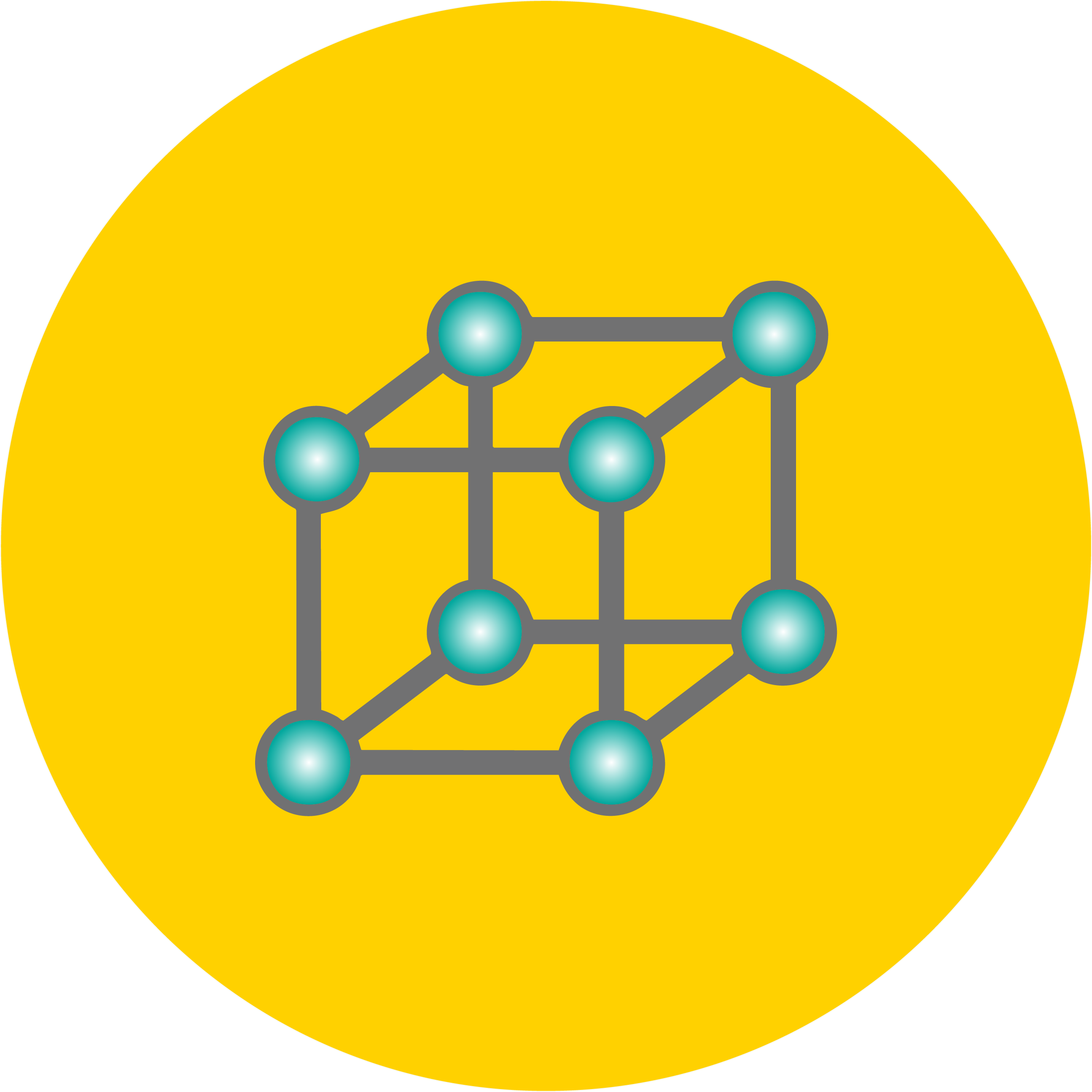
User Input: chemical composition of chosen material
Model output: predicted critical temperature of superconductivity
Licence: MIT
Semiconducting Materials
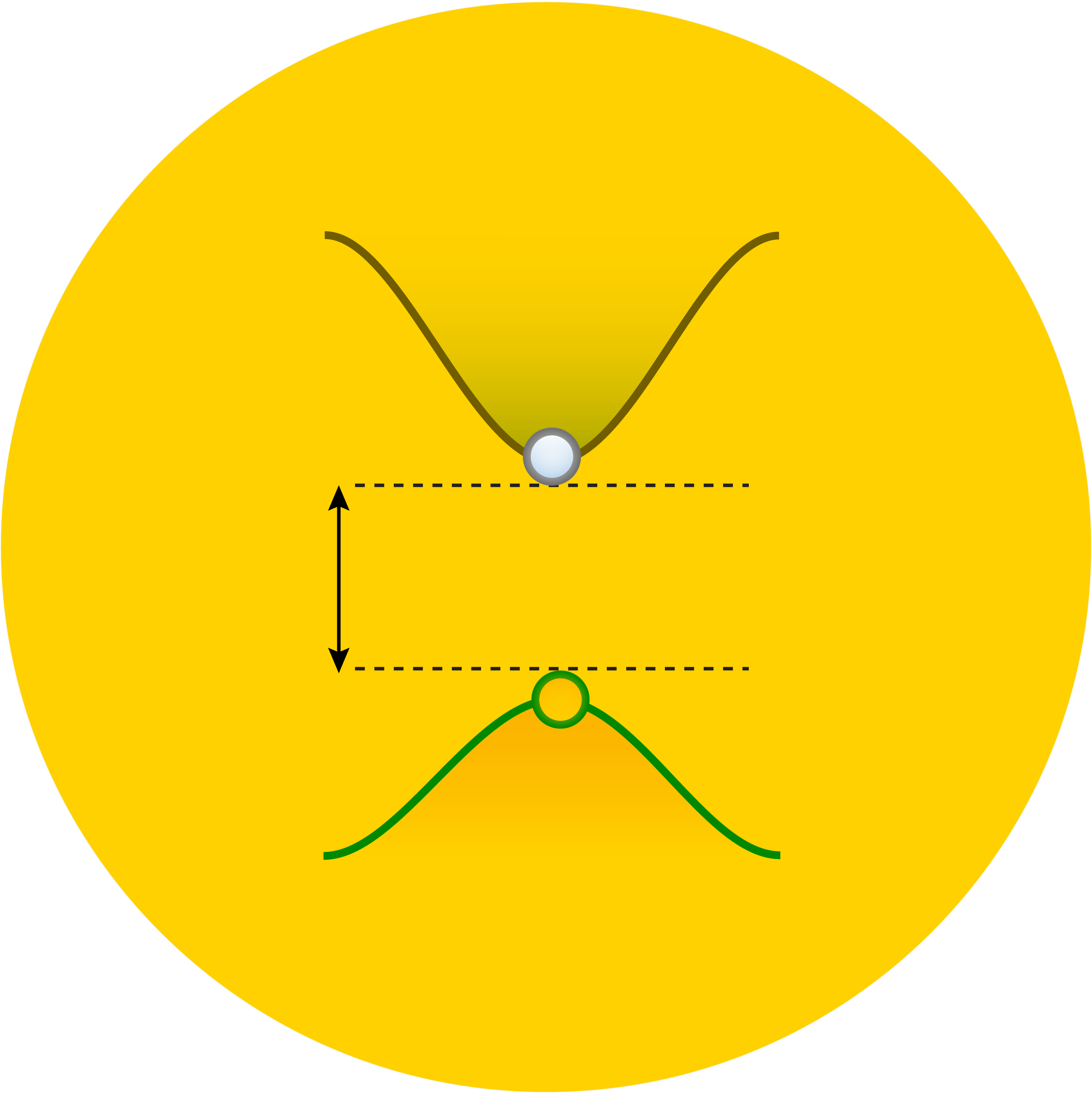
User Input: chemical composition of chosen material
Model Output: band-gap energy prediction
License: MIT
High-Entropy Alloys
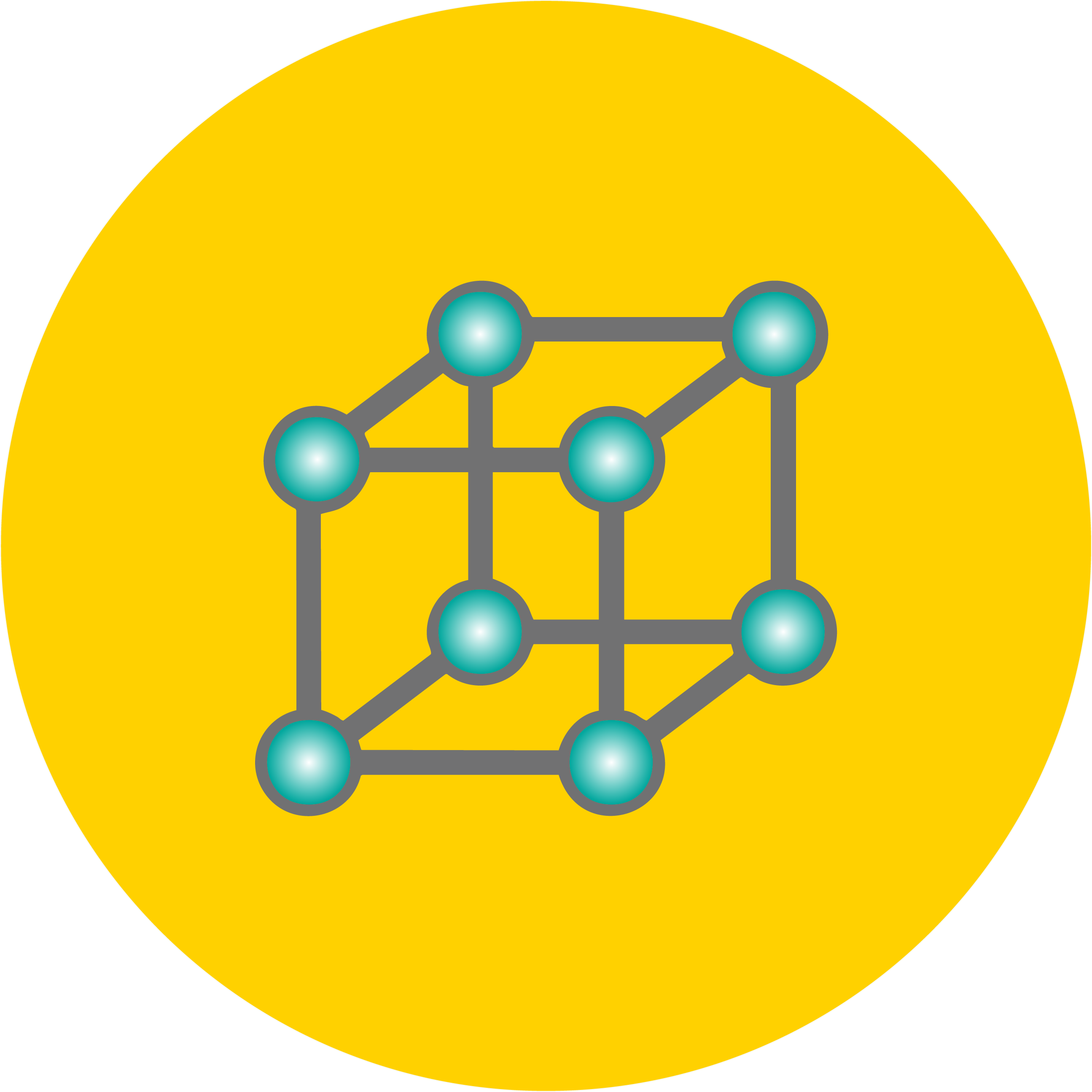
User Input: chemical composition of chosen material
Model Output: prediction of various properties that include: elastic constants, Young’s modulus, shear modulus, Wigner–Seitz radius, formation enthalpy, total energy, Zener anisotropy, Pugh ratio, Poisson ratio, universal anisotropy.
Licence: MIT
Amorphous Metallic Alloys
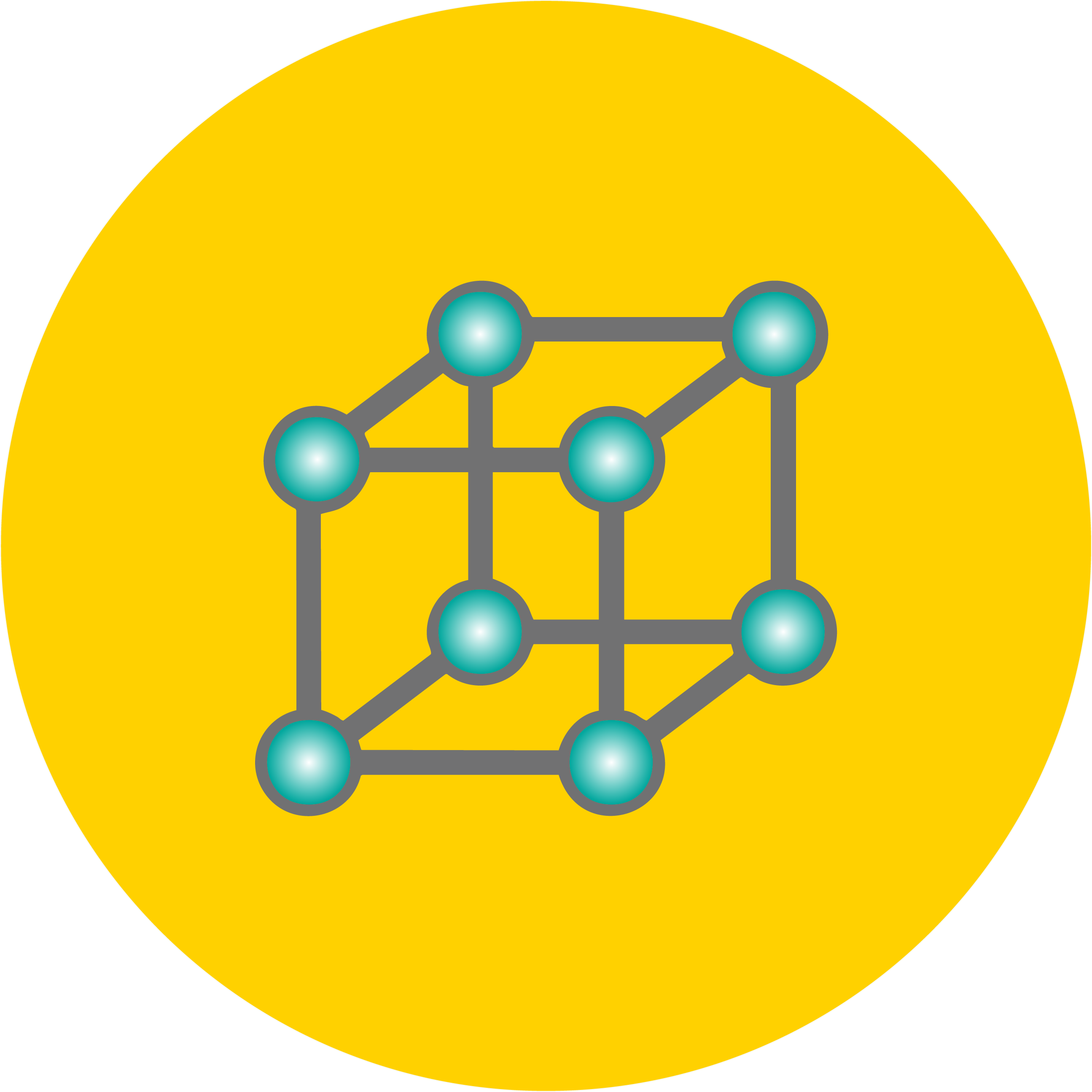
User Input: chemical composition of chosen material
Model Output: prediction of their glass forming ability and the most significant material features that govern this property
License: MIT