Library
The following resources are courtesy of: University of Cambridge Royce Partner. Digital library assembly and maintenance funded via the EPSRC AI Hubs, AIchemy [aichemy.org] (EP/Y028775/1, EP/Y028759/1), APRIL [april.ac.uk] (EP/Y029763/1) and Royce (EP/…)
Language Models for Batteries (BatteryBERT)
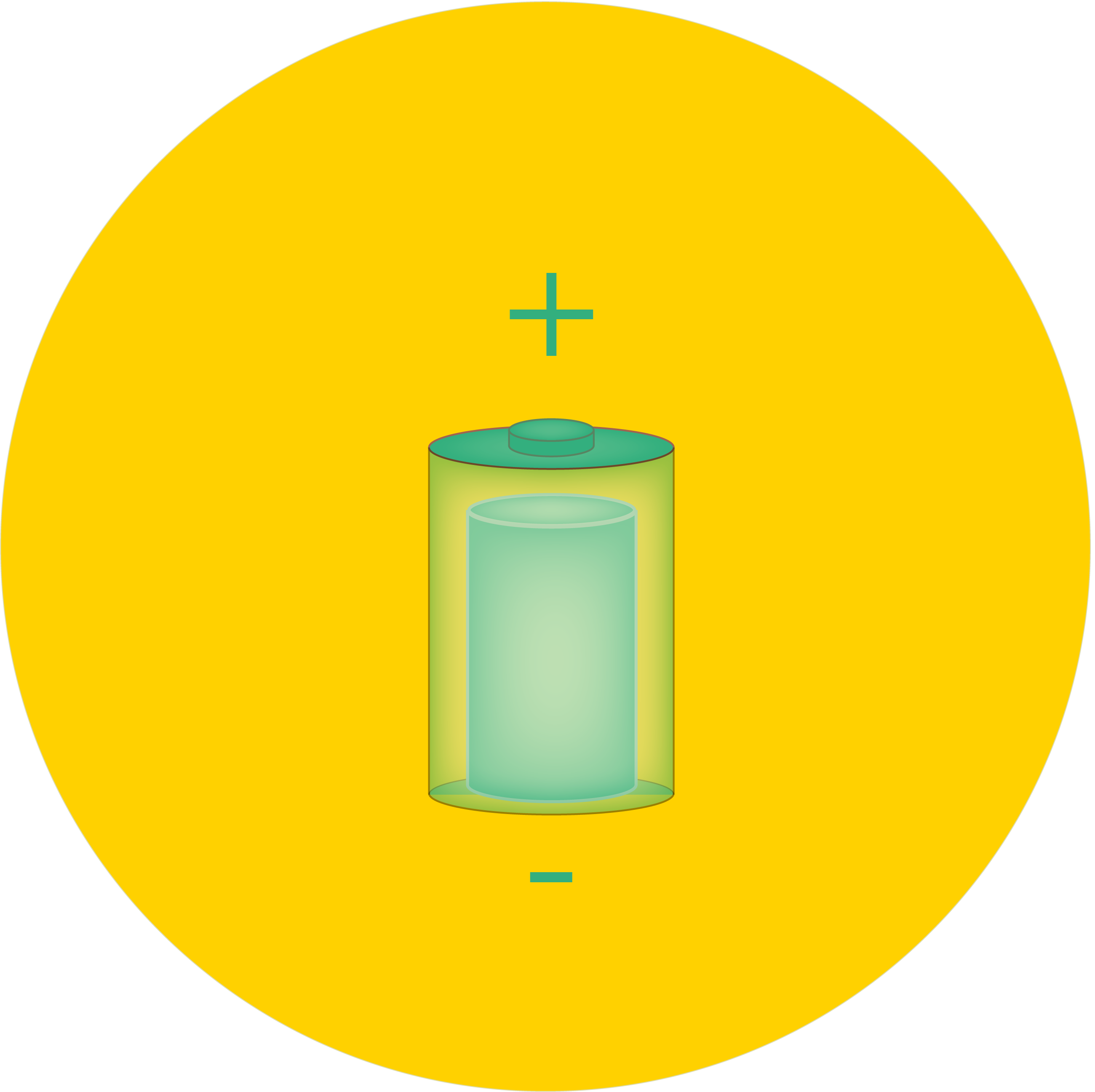
Language Models for Batteries (BatteryBERT)
Description: Trained using bidirectional encoder representations from transformers (BERT) architecture and the corpus of battery-rich academic papers that was used to create a dataset of 210K records about battery devices.
Capabilities:
(a) question-answering module that assigns battery device data to anode, cathode or electrolyte materials information.
(b) Document classifier that identifies papers about batteries.
License: apache-2.0/MIT
Language Models for Mechanical Engineering (MechBERT)
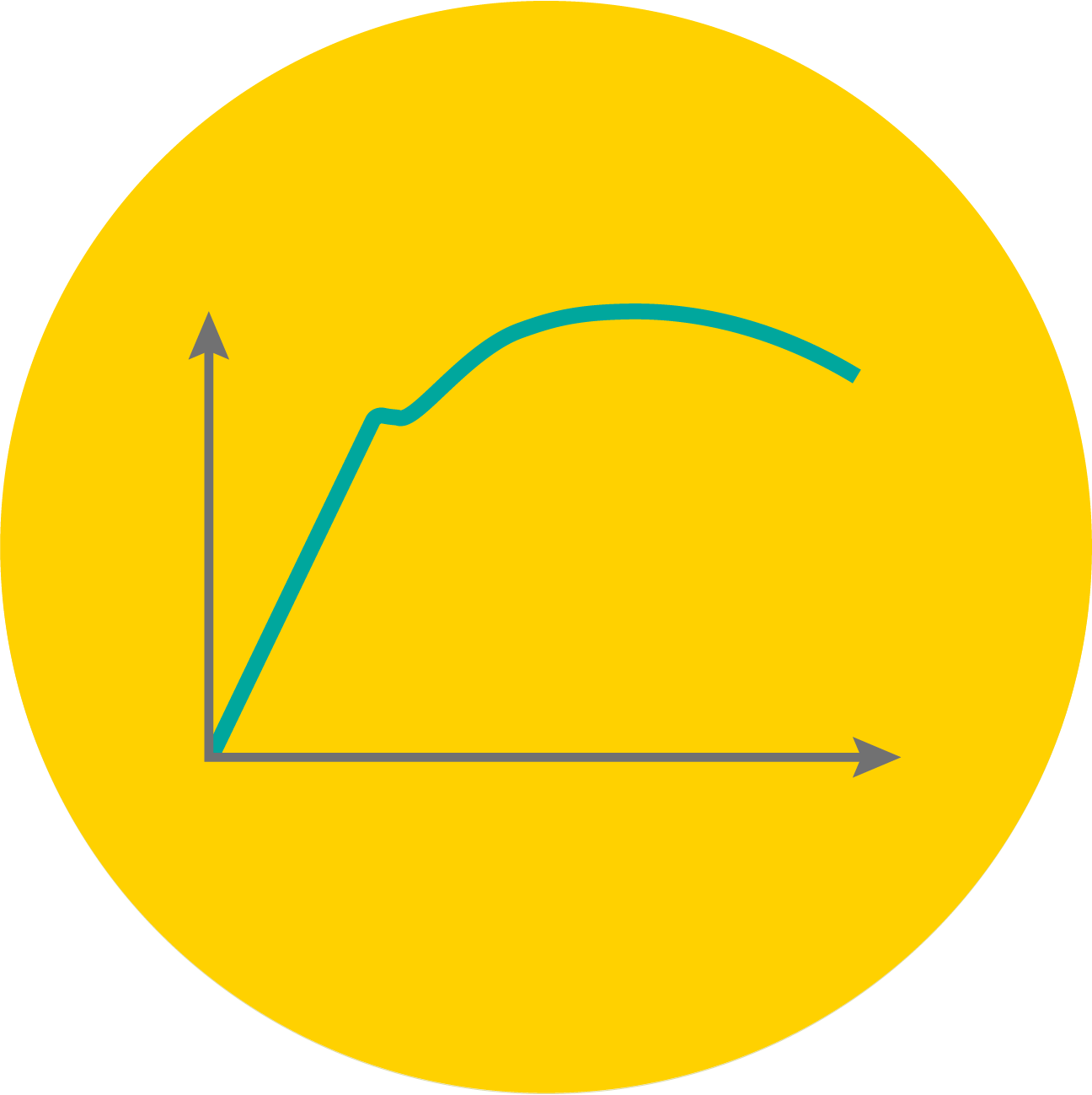
Language Models for Mechanical Engineering (MechBERT)
Description: Trained using the BERT architecture and the corpus of academic papers that was used to generate a database of 720K records about stress-strain engineering properties.
Capabilities: Question-answering tasks about stress-strain engineering.
License: GPL-3.0/MIT/MIT
Language Models for Photocatalysis Applications to Water Splitting (photocatalysisBERT)
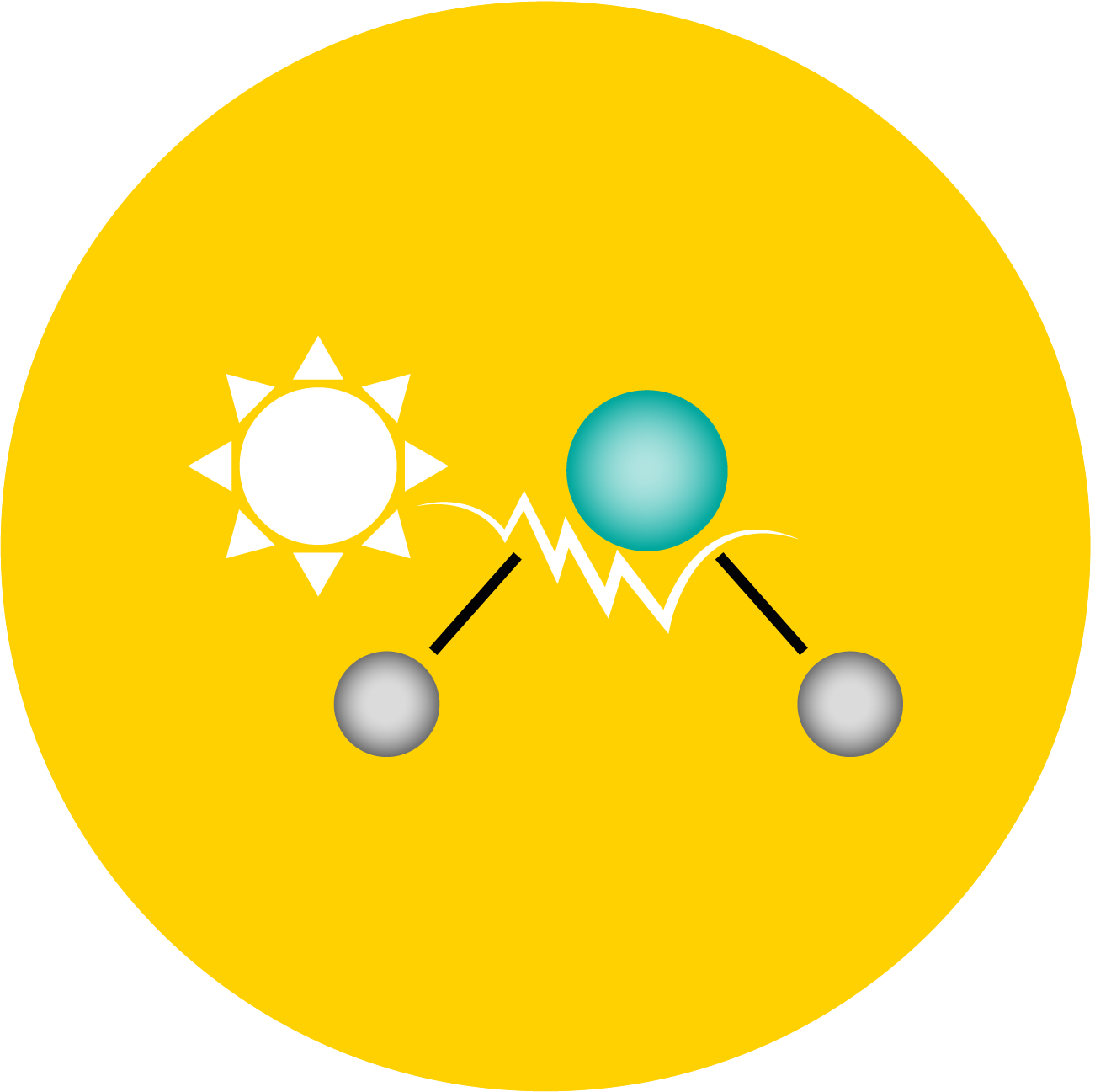
Language Models for Photocatalysis Applications to Water Splitting (photocatalysisBERT)
Description: Trained using the BERT architecture and the corpus of academic papers that was used to create a dataset of 16K records about photocatalysis for water splitting applications.
Capabilities: Multi-turn question-answering tasks.
License: MIT
Language Model for the Physical Sciences (PhysicalSciencesBERT)
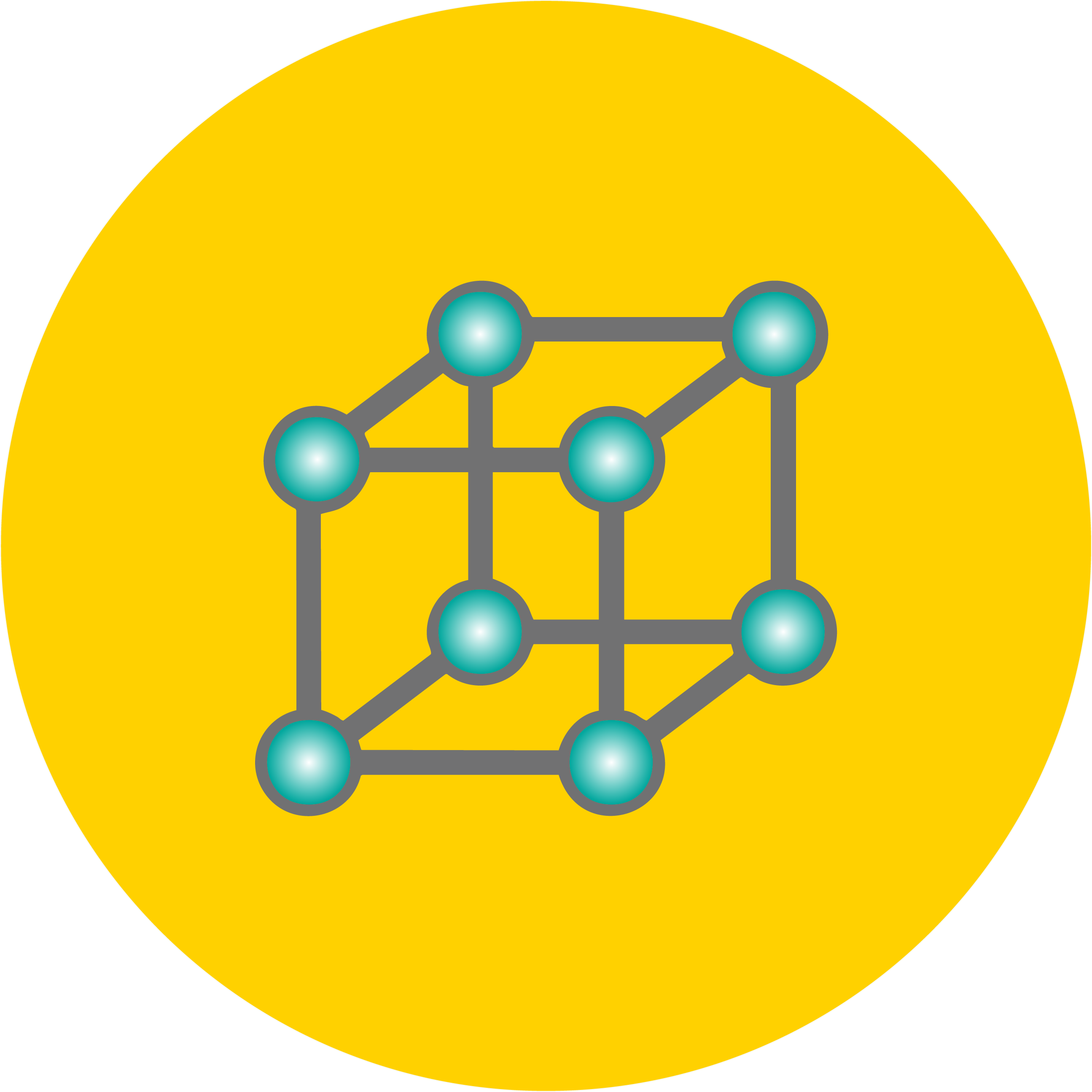
Language Model for the Physical Sciences (PhysicalSciencesBERT)
Description: Trained using the BERT architecture and a 57 GB subset of the corpus of academic papers that was used to create the S2ORC dataset which had been filtered on the subject areas: physics, chemistry and materials science.
Capabilities: Multi-turn question-answering tasks.
License: MIT
Language Models for Optoelectronics (OE-BERT, OE-ALBERT, OE-RoBERTa)
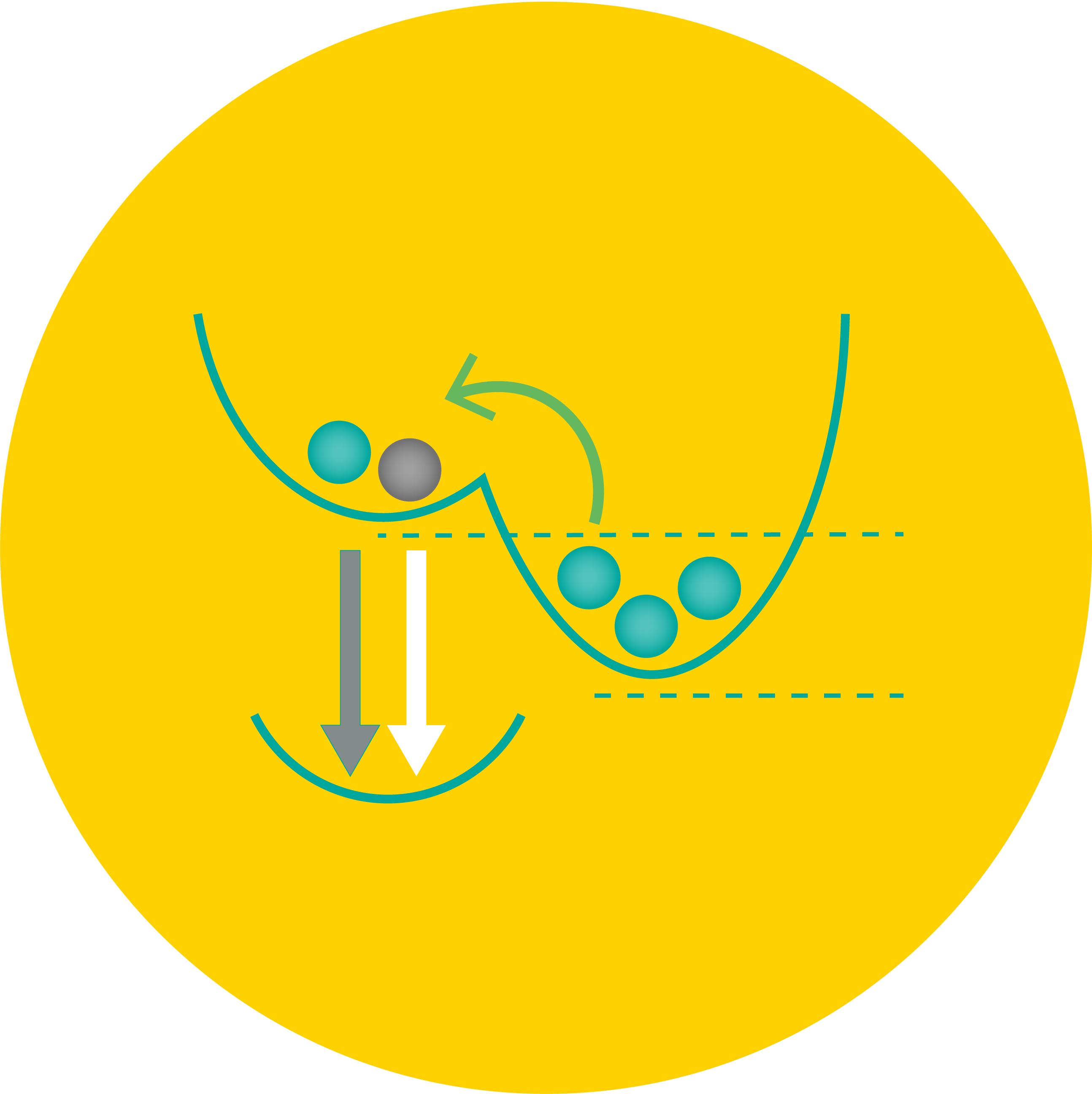
Language Models for Optoelectronics (OE-BERT, OE-ALBERT, OE-RoBERTa)
Description: Trained using the BERT, ALBERT and RoBERTa architectures and a corpus of academic papers about optoelectronic materials that comprised 5.7 GB tokens of text.
Capabilities:
(a) extractive question-answering tasks.
(b) abstract-classification tasks.
(c) text-embedding tasks.
License: CC-BY-4.0
Language Models for Optics (OpticalBERT)
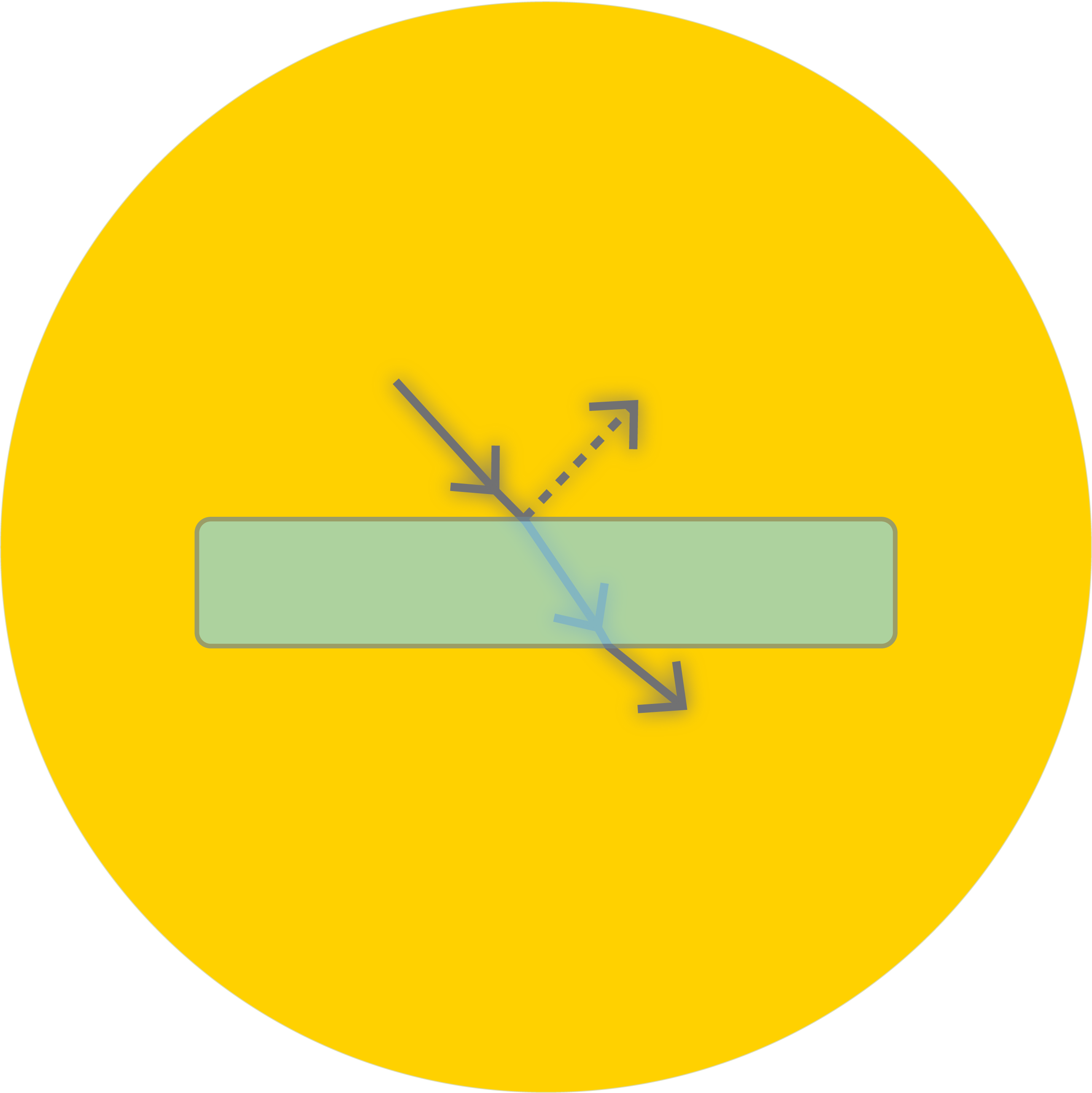
Language Models for Optics (OpticalBERT)
Description: Trained using the BERT architecture and the corpus of academic papers about optical properties that comprised 2.92 B tokens of text.
Capabilities:
(a) extractive question-answering tasks.
(b) abstract classification tasks.
(c) chemical named entity recognition.
License: CC-BY-4.0
Note: The OpticalTableSQA question-answering tool to process tables was also made in this work by fine-tuning the Tapas model.
Language Models for Photovoltaic Solar Cells
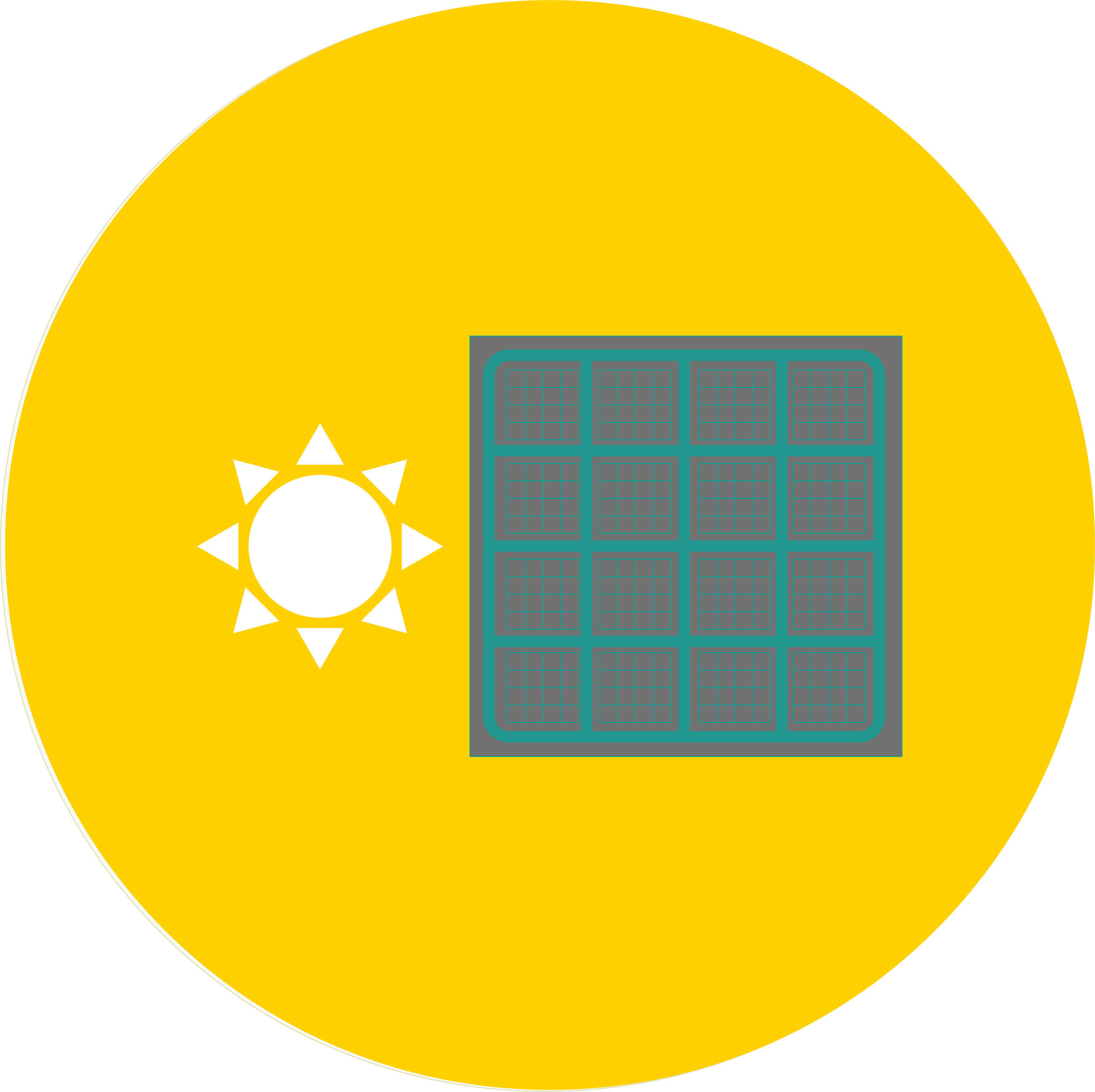
Language Models for Photovoltaic Solar Cells
Description: Trained using the BERT architecture and the corpus of academic papers that comprised 900.8 B tokens of text. These models were fine tuned using an dataset of 42,882 question-answer pairs about solar-cell properties (SCQA). This dataset was auto-generated using a custom algorithm that sourced its photovoltaic text from a database that was generated using the ChemDataExtractor tool.
Capabilities: Extractive question-answering tasks.
License: CC-BY-4.0